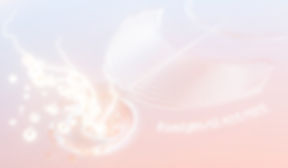
Acoustic spectroscopy
The core of every TrafoStick sold by Passerro is the smart deep learning algorithm. Based on and developed through a massive evaluation of more than nineteen thousand, in laboratories analyzed, multivariant insulating oil samples of over 3500 power-transformers from all over Europe (including Germany, Italy, Austria and Switzerland).
One of the inherent difficulties with multivariate statistics is the problem of visualizing data that has many variables (including age, acidity, temperature, water content, and breakdown voltage). For example, a function diagram displays a diagram of the relationship between two variables. However, when there are more than three variables, it is more difficult to visualize their relationships.
Fortunately, in datasets with many variables, groups of variables often move together. One reason for this is that there may be more than one variable measuring the same drive principle that determines the behavior of the system, in our case the value of the breakdown voltage. In many systems there are few such drivers. But an abundance of instruments allows you to measure dozens of system variables. When this happens, you can take advantage of this redundancy of information. You can simplify the problem by replacing a group of variables with a single new variable.

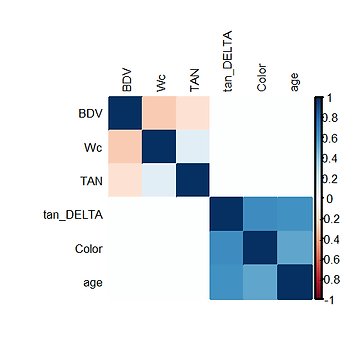
Principal component analysis is a quantitatively rigorous method to achieve this simplification. The method creates a new set of variables called principal components. The correlation graph shows that two variables play a crucial role in the system of breakdown voltage. The total acid number (TAN) and water content (Wc).

The graphic above shows the distribution between TAN and Wc of all data sets available to us. It can be seen that only a certain behavior of the samples can be observed in transformers in field use. The respective parameters only assume values within fixed limits. This knowledge was the base for the formation of the mathematical model for the calculation of the breakdown voltage.
The model is based on the actual behavior of the oil, which is caused by years of everyday operation in transformers.

The logarithmic representation of the sample distribution shows the limit of the resolution of the laboratory data collection (titration). The isolines drawn always describe the 10% gradation of the number of samples.
The main goal was to find a method that allows continuous TAN & WC measurement. Inspired by the work of Howells & Norton and Hlavay & Guilbault , we were looking for a way to use ultrasound directly or as an acoustic resonator to solve the problem of BDV estimation through water and acid detection in an online device. With an aluminum-coated piezoelectric resonator we found out that the ultrasonic relaxation process, as it is described by HOKneser , related to the energy transfer between translational and oscillating degrees of freedom, for the measurement of a kind of acoustic imbalance [AcDis] and further directly for an estimation of the water v. TAN balance can be used.

Spectral function of the piezoelectric resonator at its first resonance point
We use the resonator quality factor ( Q factor ) to calculate AcDis. By definition, the Q-factor is a dimensionless parameter that describes how underdamped an oscillator or resonator is and that characterizes the bandwidth of a resonator relative to its center frequency.
The method is based on the basic idea that the combination of acoustic spectroscopy and the acquisition of an RS value of the transformer oil is sufficient for a sufficiently precise determination of the condition of a transformer oil.
There is a great need for a device in high-voltage technology which ensures a quick, reliable and precise determination of the condition of the insulation oil in order to avoid unnecessary and cost-intensive oil changes. This task is solved in a surprisingly simple but effective way by a TrafoStick for monitoring the condition of a transformer oil.